Caching slow functions in Python
Suppose you wrote a function that returns the user's email:
def get_user_email(user_id):
user = find_by_id(user_id)
return user["email"]
But there is a problem. find_by_id()
calls a terribly slow legacy system:
def find_by_id(user_id):
# simulate a slow network request,
# returning a user by their id
time.sleep(1)
return { "email": "..." }
100 calls for get_user_email(42)
result in 100 slow requests. A single one should be quite enough, so let's attach a simple cache:
cache = {}
def get_user_email(user_id):
if user_id not in cache:
user = find_by_id(user_id)
cache[user_id] = user["email"]
return cache[user_id]
Nothing too complicated (apart from the issue of cache expiration, let's not touch it). But imagine that there are a lot of slow functions, and you have to implement caching for every one of them. Not too inspiring.
Fortunately, there is an @lru_cache
decorator in the functools
module. That's what we need. Add one line to the original function, and be done with it:
@functools.lru_cache(maxsize=256)
def get_user_email(user_id):
user = find_by_id(user_id)
return user["email"]
Now repeated calls to get_user_email()
with the same user_id
return the cached result without requesting find_by_id()
.
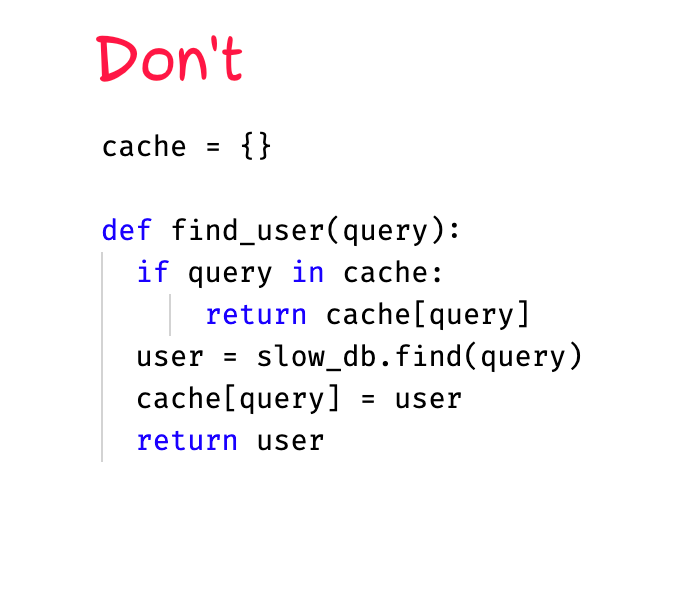
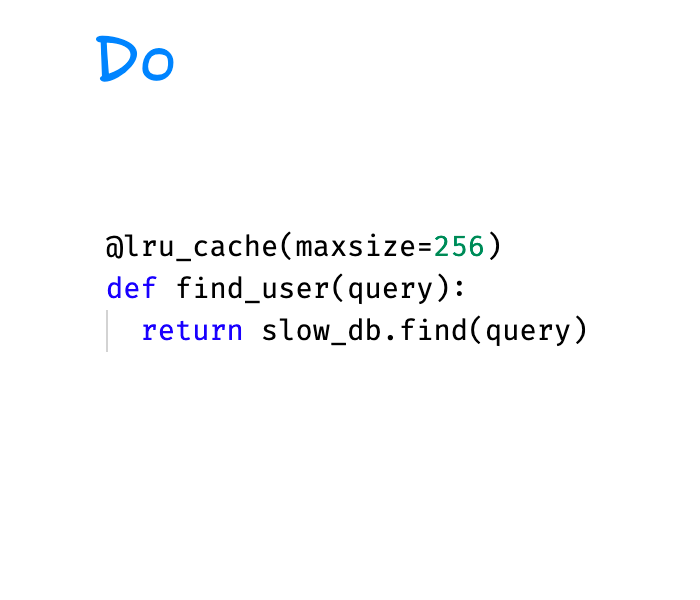
@lru_cache
automatically evicts old entries from the cache when there are more than maxsize
entries. So the cache won't eat up all the memory.
Python 3.9 received another decorator — @functools.cache
. It's similar to @lru_cache
, only without the cache entry limit (which makes it work a little faster).
One can manage the cache — view hits/misses stats or clean it:
# cache management
stats = get_user_email.cache_info()
print(stats)
# CacheInfo(hits=2, misses=3, maxsize=256, currsize=3)
get_user_email.cache_clear()
# CacheInfo(hits=0, misses=0, maxsize=256, currsize=0)
The cache works in-process and will die with it. So if you need something more scalable, look at Redis or other external cache.
★ Subscribe to keep up with new posts.